Introduction
Data quality is a critical pillar in the data governance architecture, and it is critical to an organization’s ability to achieve defined governance criteria.
While both exist as standalone models, the proper deployment of data quality and data governance structures has the potential to build a symbiotic system that eventually supports an organization’s strategic goals and informs decision-making.
Data assets, like physical assets like machinery, equipment, and real estate, require maintenance and management in the same way that physical assets like machinery, equipment, and real estate do.
When discussing the usefulness of business data, we frequently hear the terms “data governance” and “data quality” used interchangeably. In truth, they are two independent disciplines, but they are inextricably linked in a symbiotic connection in which each feeds off the other.
What is Data Quality?
Data quality guarantees that vital information is accurate, full, and consistent throughout the enterprise’s different domains. For a variety of reasons, accuracy diminishes. When information changes, data may become erroneous simply due to being out of date. This is especially frequent with consumer data, which, on average, becomes less accurate with time.
Accuracy may also be the consequence of input mistakes or the gathering of low-quality data from an outside source. Incomplete information might also lead to poor data quality.
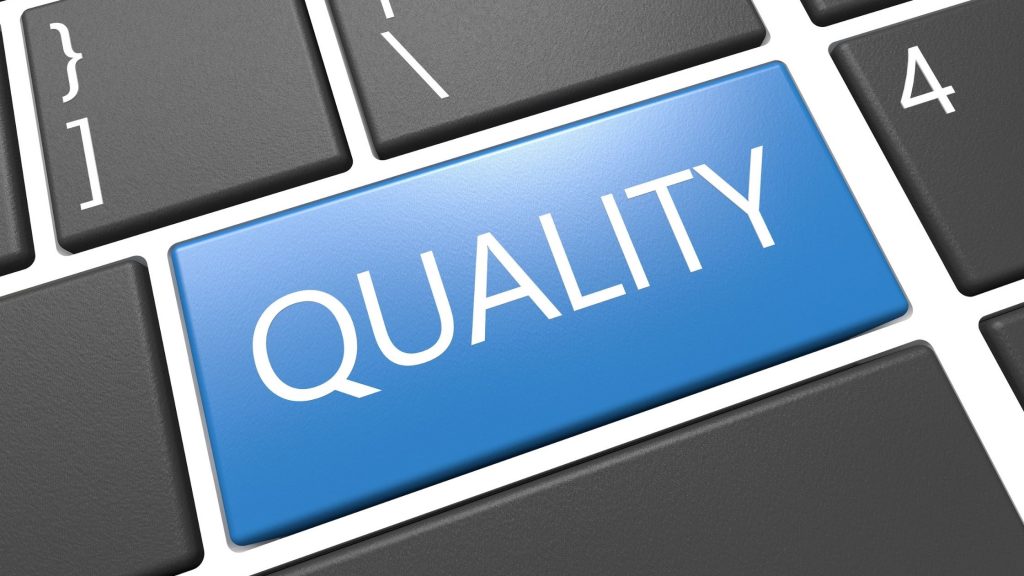
Again, this might be due to a clerical error during data input, or it could occur when new data pieces are introduced and no provision is made for updating current information.
What is Data Governance?
Data governance is closely connected to, but fundamentally distinct from, quality. Data governance is the management of an organization’s information. It is a procedure that determines who has the authority to see and use information. It standardized how this information is gathered, kept, evaluated, and disseminated for a specified purpose.
Data governance is a broad concept that incorporates various components. These elements are usually defined in a data management strategy or a data standard.
Role of Data Quality in Data Governance
- Data governance and Data quality are inextricably linked. Each is necessary for the other, and businesses that want to derive substantial value from their data assets must keep both in mind. Both need continual work; neither is a “one-and-done” affair.
- Information consistency across several databases within an organization can potentially be a cause of quality issues. Mismatched addresses, for example, in CRM vs. ERP, may result in shipments being delivered to the incorrect destination. Duplicate and out-of-date records are quite prevalent. They cost businesses millions of dollars each year, for example, by squandering money by repeatedly reaching out to the same consumers or marketing to firms that no longer exist.
- To achieve data quality, established methods should be in place to monitor data integrity and identify anomalies or errors in the data being processed. A robust data quality system lays up clear paths for resolving issues, implementing changes, and maintaining ongoing monitoring.
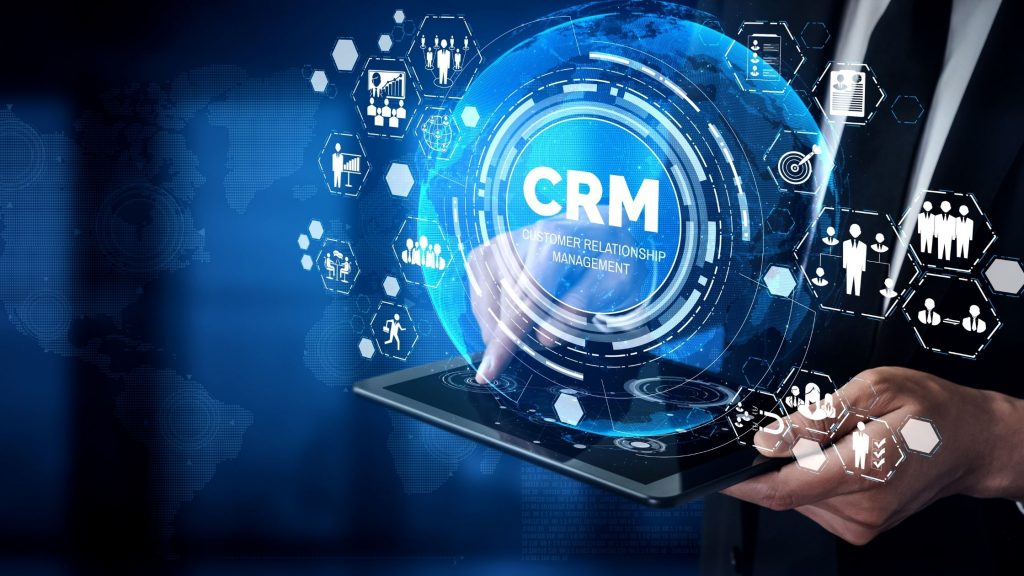
- What is deemed excellent data is also influenced by the end user. If the information provided is unusable, regardless of its correctness and completeness, it may be termed low quality since it does not match the demands of the end user.
- In certain ways, data quality is almost a component of governance. After all, it’s difficult to envisage successful data governance without taking care of data quality. However, the opposite is also true.
- Without an appropriate governance system in place, it is impossible to reach meaningful levels of data quality. This is especially true at a larger scale.
- We can envisage an endeavor to increase quality in the framework of a well-defined domain in smaller, more concentrated situations. Cleaning up the CRM database, for example, might be done in relative isolation.
- However, in order to perform a good job, data governance and data quality must be viewed as two sides of the same coin.
Takeaway
If your business is just starting with data governance and data quality initiatives, following the steps below will help your program succeed. These methods are also applicable to companies attempting to improve existing programs.
- Be willing to start small and work your way up. As with many other pursuits, there is value in beginning with a small project and learning from your early attempts. Prioritizing the data sources and integration points that are most relevant to the company is critical. By demonstrating success with a smaller project at first, you may demonstrate the business benefit of data quality and governance activities, get further support within the company, and position yourself for future success.
- Collaborate. Not everyone in the organization will immediately see the importance of governance activities. That doesn’t mean you shouldn’t involve them in the process when you can. You will be better positioned to develop momentum in the future if you include multiple ideas and goals.
- Analyze your outcomes. Data quality and governance generate measurable business benefits. Seek out colleagues from different departments, learn about their goals and pain areas, and shape your data activities around chances to provide business value. Your strategy should include how you will measure your results so that you may improve on your previous efforts with the following iteration.
Finally, both collaborate to guarantee that the information provided to stakeholders throughout the company is reliable, safe, and accessible when needed. Business leaders can guarantee that their businesses are well-positioned to maximize the value of their data assets by constructing a relevant strategy around these two pillars.