Introduction
Good data management methods are critical for ensuring that research data is of good quality, easily found, and accessible. You may then exchange data to ensure its long-term sustainability and accessibility for new research and policy, as well as to duplicate and validate existing research and policy. It is critical that researchers apply these methods to their work with all forms of data, whether huge (complex) or little (curatable). In this blog post, we will learn about data curation. Furthermore, we will investigate many other benefits that data curation will bring to the big data table.
Data Curation
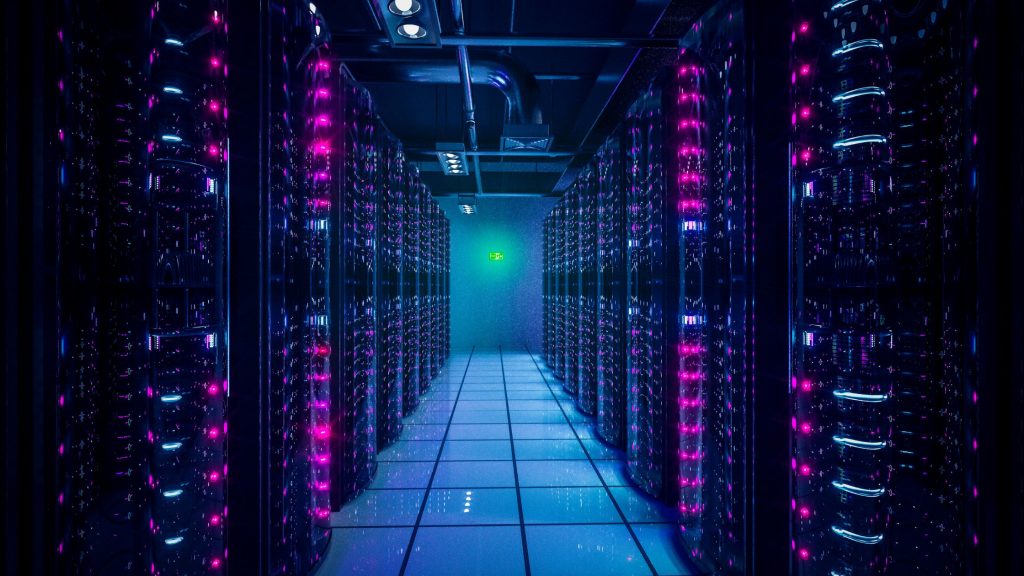
Curation is the end-to-end process of producing good data by identifying and developing resources with long-term worth. It mostly refers to the management of data throughout its lifespan, from creation and initial storage until the moment when it is stored for future research and analysis, or becomes obsolete and is removed, in information technology. The purpose of enterprise data curation is twofold: to maintain compliance and to ensure that data may be recovered for future research or usage.
Role of Data Curation in Big Data
In reality, data curation is more concerned with preserving and managing metadata than with the database itself, and a big portion of the data curation process focuses around ingesting metadata such as schema, table and column popularity, usage popularity, and top joins/filters/queries. Data curators not only generate, manage, and preserve data, but they may also establish best practices for engaging with that data. They frequently show data in a graphical style, such as a chart, dashboard, or report.
The “data set” is the starting point for data curation. These data sets are the building blocks of data curation. The data curator’s task is to determine which of these data sets are the most helpful or relevant. It is also critical to be able to convey the facts in an effective manner. While certain general guidelines and best practices apply, the data curator must make an informed judgment about which data assets to employ.
Before data is trusted, it must be understood in its context. To establish the relevance of data assets, data curation employs current taste arbiters such as lists, popularity rankings, annotations, relevance feeds, comments, articles, and the upvoting or downvoting of data assets.
Importance of Data Curation
Firms invest substantially in big data analytics — $44 billion in 2014 alone, according to Gartner — yet studies reveal that most organizations only use around 10% of the data they acquire, data that is distributed across the enterprise in silos and from various sources. With data quantities increasing at an exponential rate, as well as the diversity and heterogeneity of data sources, preparing the data for analysis has become an expensive and time-consuming procedure. Before various analytics tools may leverage many data sets from diverse sources, they must first be classified and linked. Duplicate data and missing fields must be removed, misspellings corrected, columns divided or reshaped, and data supplemented with data from other or third-party sources to offer greater context.
- Dealing with Data Swamps
A Data Lake strategy enables users to quickly access raw data, analyze numerous data properties at the same time, and ask ambiguous business-driven questions. However, Data Lakes may devolve into Data Swamps, where locating business value becomes akin to searching for the Holy Grail. Such Data bogs could as well be a Data cemetery. Data curation helps keep your data lakes from becoming data yards.
- Effective Machine Learning
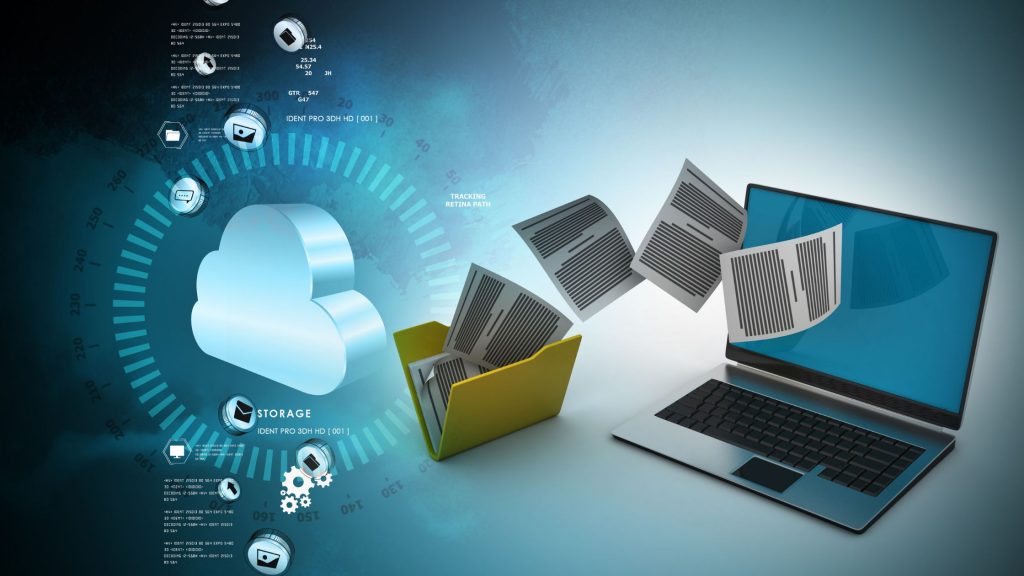
The understanding of the consumer market via algorithms has advanced significantly. AI is composed of “neural networks” that interact and can identify patterns using Deep Learning. Humans, on the other hand, must intervene, at least initially, to lead algorithmic behaviour toward effective learning. Curations are places where people can apply their expertise to what the computer has automated. As a consequence, enterprises are better prepared for insights by preparing for intelligent self-service procedures.
- Ensuring Data Quality
Data Curators clean and take activities to guarantee the long-term preservation and conservation of the authoritative nature of digital artifacts.
Summing Up
Data sets are reusable components; anybody undertaking analysis should share and expect their data sets to be reused. Reusability is essential for self-service at scale. Many companies have already embraced this strategy for data gathering and distribution for re-use, allowing every user to become a curator of data expertise and resulting in increased productivity.
Data curation looks at how data is used, concentrating on how context, story, and meaning may be gathered around a reusable data collection. It fosters data trust by measuring the social network and social ties between data consumers. Curation goes beyond data documentation to build confidence in data throughout the company by utilizing lists, popularity rankings, annotations, relevance feeds, comments, articles, and the upvoting or downvoting of data assets.