Data curation is a critical component of data management. Data curation is the process of gathering, organizing, and keeping data. It enables businesses to keep sustainable and accessible data to share and apply self-service analytics. Data-driven insights are critical because data-driven sales techniques help businesses increase sales productivity by 20%.
However, businesses only evaluate 12% of their data on average. As a result, data scientists are motivated to learn more about data curation techniques to curate additional datasets and metadata.
What is Data Curation?
Data curation efforts (collecting, wrangling, and preservation) result in curated datasets. The primary goal is to create FAIR (Findable, Accessible, Interoperable, and Reusable) and analytic data. Ultimately, the goal is to maximize the value of data, as evaluated by the developing field of infonomics.
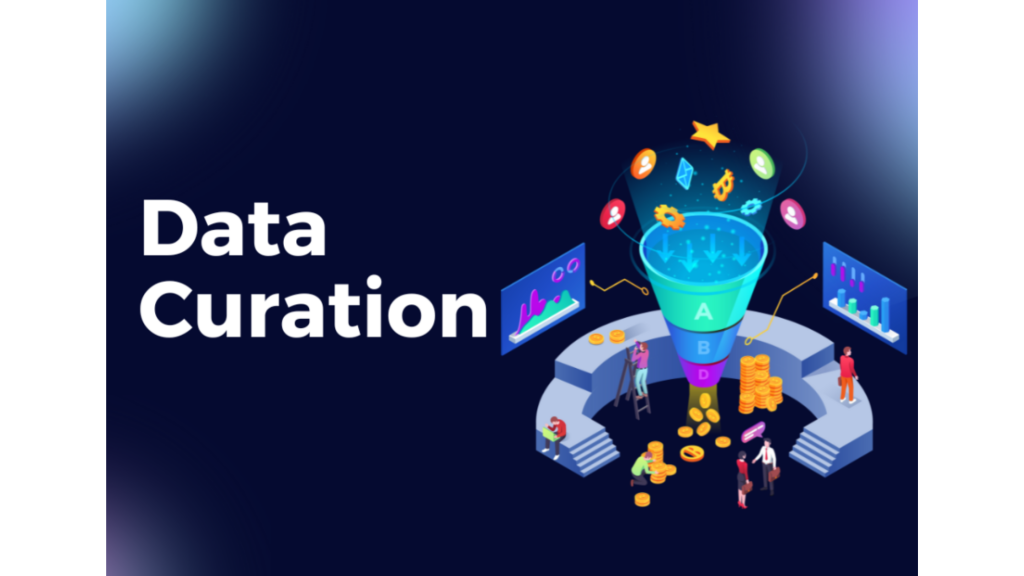
What steps are involved in data curation?
Data curation consists of several processes, beginning with data collection and progressing through preprocessing, cleaning, and augmentation. Here’s the step-by-step breakdown:
- Data collection
This is the initial stage in gathering data from multiple sources. Databases, websites, Internet of Things devices, social media, and other sources can all be used. Data gathered might be either unstructured (such as text or photographs) or organized (such as CSV files or databases).
- Data cleaning
Following collection, the data is cleansed. This includes dealing with missing numbers, removing duplicates, addressing outliers, and correcting discrepancies. Cleaning guarantees the data’s quality and correctness, making it suitable for the next processes.
- Data annotation
Depending on the machine learning job, data may require annotation. For example, in image recognition tasks, photographs are tagged to identify what item is there. Text can be annotated to show elements of speech or sentiment in natural language processing jobs. Annotation facilitates supervised learning, which is when a model learns from instances.
- Data transformation
Why is data transformation important? The cleaned and annotated data may need to be converted to a format appropriate for machine learning techniques. This might include categorical data, one-hot encoding, numerical data normalization or standardization, or even text conversion to number sequences.
- Data integration
If data is acquired from numerous sources, it must be integrated in a consistent and relevant manner. This might include aligning data based on timestamps or integrating datasets using shared IDs. Discover why data integration is vital.
- Data maintenance
Over time, the data may need to be updated or supplemented with new information. Maintaining the dataset guarantees that it is still relevant and valuable for continuing machine learning operations.
Data curation is to guarantee that the data utilized in machine learning activities is as accurate, consistent, and high-quality as possible. Well-curated data leads to more effective machine learning models, which improve their performance and generalizability to new data.
Why is Data Curation Important?
Examples of erroneous datasets include incorrect information, knowledge gaps, and incorrect recommendations. The datasets can be:
- Some AI employed for picture identification shows gender and racial prejudice.
- Inaccurate, untrustworthy, or wrongly presented
- Error-prone or ambiguous.
The lack of treated or curated raw datasets diminishes feature quality while also limiting data creation and applications. Therefore, firms may use data curation for:
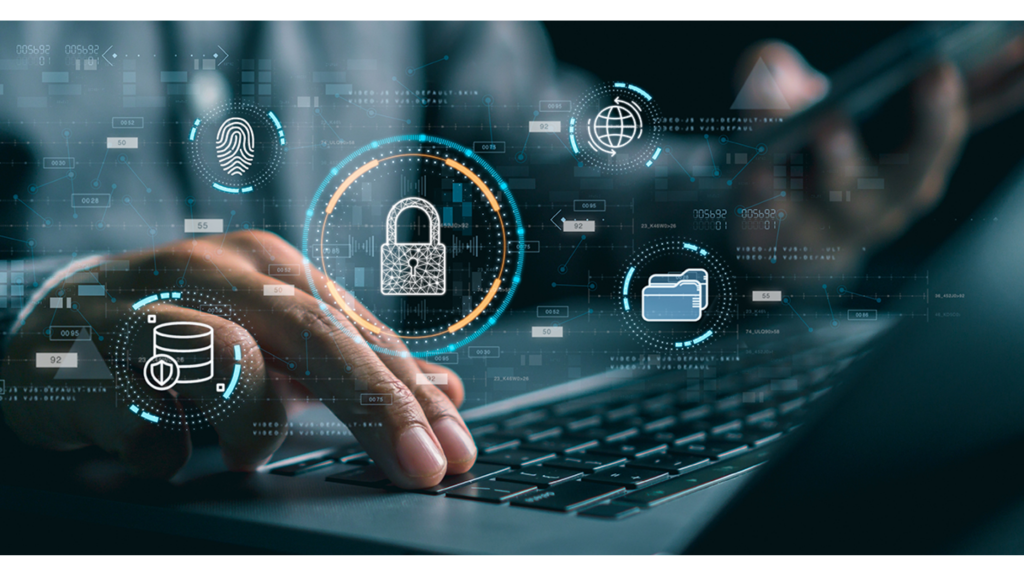
- Machine Learning
Data curation is used to prepare training data for machine learning (ML) and artificial intelligence (AI) applications. Data curation strategies are used to properly identify and categorize training data, making it trustworthy, unbiased, and machine-readable.
- Data Quality
Data curation organizes, characterizes, cleans, and maintains data, allowing business analysts to work with accurate information in the long run. Without data curation, data would be difficult to obtain, handle, and understand. This would avoid the formation of data swamps. A data swamp refers to circumstances in which data storage and access are not properly controlled, resulting in useless data. Data curation allows for data separation and the retention of high-quality data in the lake. Thus, it may be used to recover data swamps.
What are the problems involved in data curation?
Data curation may be an expensive and difficult procedure when dealing with large amounts of unstructured data. In such cases, the data creator examines multiple data curation methodologies and manages a large number of diverse data sets.
Furthermore, data has not been collected following its intended use for decades. Organizations were unsure how to incorporate the data into their strategic decision-making. Before data curation is implemented, companies must utilize their experience and understanding of the types of data, their worth, and why and how to use them.
Conclusion
Data curation tools improve the pre-processing stages of data management. These technologies ensure the integrity and usefulness of data. These technologies use AI and machine learning to evaluate metadata and create insights into the correct repository.
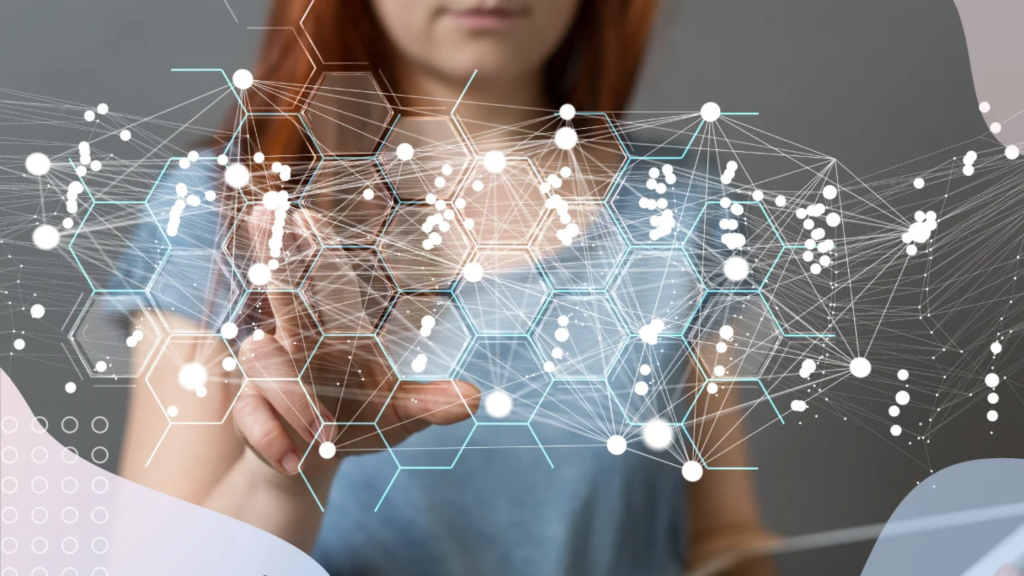
The value of data-driven decision-making cannot be overstated. As firms expand, data curators have the challenge of identifying a needle in a haystack. Implementing these best practices and collaborating with a partner like Oriental Solutions can help organize the data. Finally, investing in data expertise like Oriental Solutions for a data catalog may encourage data-savvy personnel and foster a data-driven culture in which data is the glue that drives business results.