One layer of data analytics stack is data ingestion a process where ETL process (Extract, Transform and Load) moves data from source to target storage systems. It is the process of scraping, moving the data to the central repository for further downstream cleansing processes. The process is now further enhanced by ETL tools that bring about automation, driving down costs and turnaround time!
Why is ETL process a necessity for any business?
ETL is a life-cycle of data processes for replicating data from different sources to target systems using extracting, processing, and transporting information for using in analytics, reporting, or applications. As businesses rely on data analytics for BI, reporting, and analysis, ETL is a critical process in business data management.
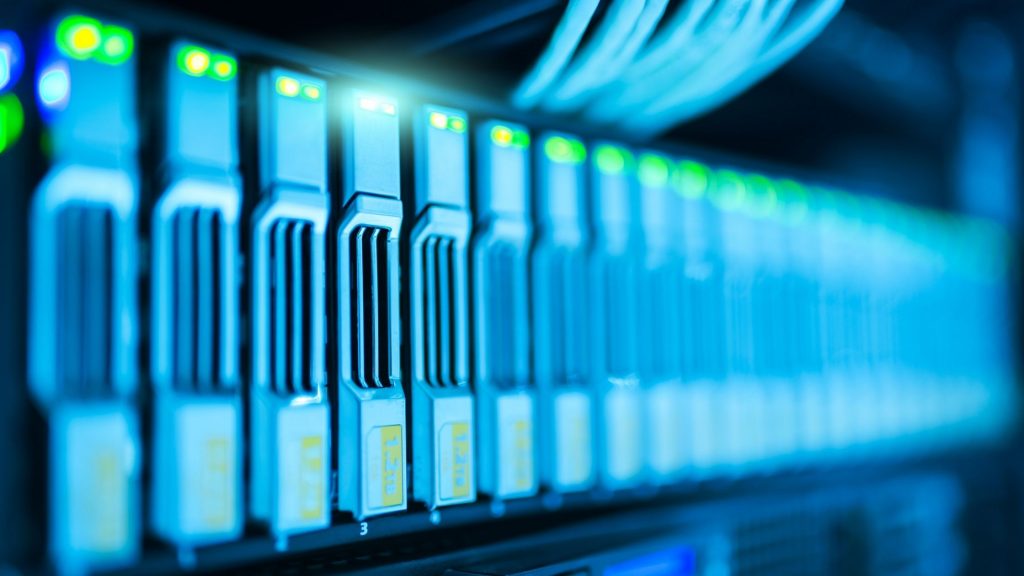
In the ETL process or data, ingestion data is moved from diverse sources to a storage medium for need-based access, use, and analysis by the organization. Such a destination could be a data warehouse, document store, data mart, digital cloud-data warehouse, or a database. The sources may be external or internal, varying from SaaS data, spreadsheets and information scraped from the internet. Since all other activities like downstream reporting and analytics need consistent, accessible data. Different architectures such as batch ingestion, streaming ingestion are adopted to optimize the data strategy.
Therefore, the type of ETL tools you will use will determine the process adopted for building the pipeline to your data warehouse.
Key benefits that ETL process brings to your business is – migration of databases, infusing transformation, data warehousing, self-service data, measure data quality and draw metadata.
Best 6 Benefits of ETL process
- Migration of databases
Data solution providers and outsourcing service providers who rely on accurate analysis of transactions or claims leverage databases. These processes transact/spotlight particular transactions from a server or cloud aiding business intelligence.
ETL primarily helps in data migration from traditional systems to digital warehouses that have a format by default. Additionally, entrepreneurs are using data from external suppliers to integrate with internal data for consolidated analysis and gain performance insights. Hence, migration of databases is an important benefit for every business.
- Infusing transformation
Data is driving businesses and transforming operations, business models. Data insights are also become the differentiators for enterprises to gain competitive edge over peers. Therefore, most businesses are engaged in scraping all forms of content available online like social media, videos, time stamps, internet of things, crowd sourced data, open sourced data and more.
Data is transforming businesses as it provides insights, outside of the traditional thought processes. There is wider scope for breakthrough thinking and transformation of businesses in unconventional pathways. Transformation is brought about by the rapid processing of data be it about trends, induction of latest technology, updating technologies and trends, innovations for an efficient and rapid turnaround space for businesses.
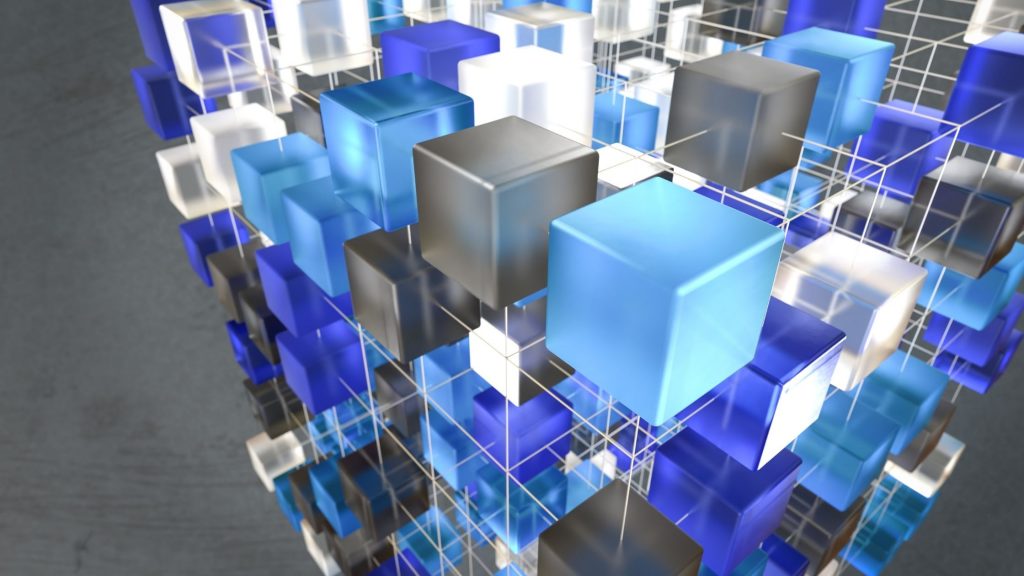
- Data storage and warehousing
Data warehouses hold cleansed data as the original data that arrives from diverse sources are converted into high-quality data that are consistently true or valid for business use. ELT process populating the warehouse and updates the warehouse, converting structured and unstructured data for the cloud/hadoop. The big data on transactions, customer interactions and operational insights, BI and ensure master data is available along with cloud data for further AI development.
The process and integrate search organizations are using tools such as extract load and transform to scale workloads. And establish data warehouse spaces in an inexpensive but efficient manner. Quality performance is easy to determine using data integration at external (such as cloud) and internal levels (such as relational data warehousing). ETL tools enhance data warehouses and impact the future of data integration.
- Self-service data
The process of extracting data from multiple resources using tools is self-service data ingestion. By adding collaboration tools, organizations can develop and maintain data warehouses, remotely or from a particular destination for specific data.
Using Graphic user Interface (GUI) users can map the flow of data in the process using drag-and-drop rules. Users can understand each step from source systems to data warehouses for data flow and logic.
- Data quality metrics
Databases are typically examined for authenticity, validity and value proposition after data science processes of cleansing, profiling and auditing is completed. Experts interpret the lineage and quality after the output is integrated with different data solutions.
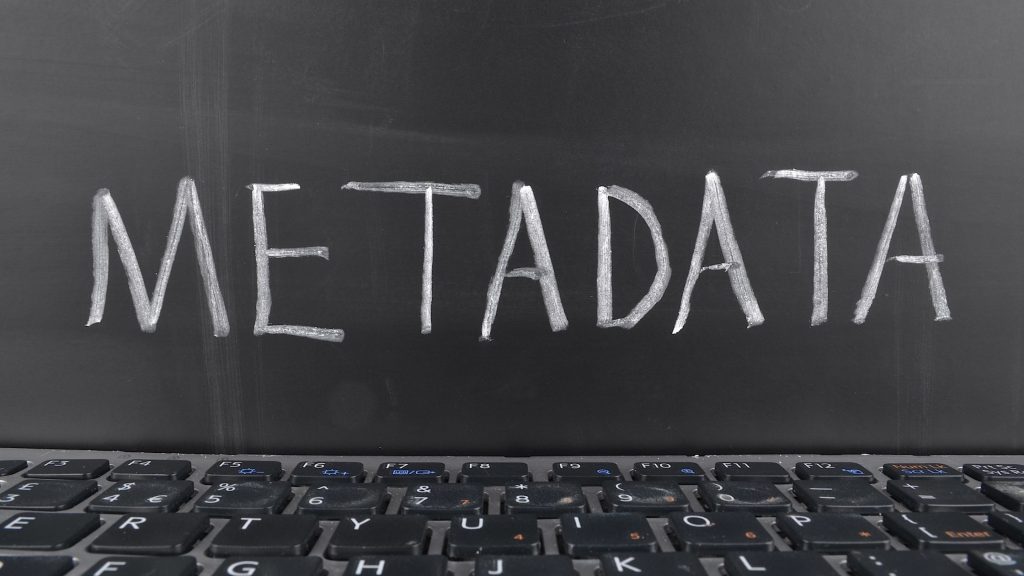
- Draw metadata
ETL tools will design complex data integration tasks to move data. Populate data warehouse for integrating data using multiple source systems. Provided metadata about the existing data can be handled to help in managing data governance tasks. And supporting data quality processes for helping teams to build and extend the warehouse.
A crucial summary of the data lineage is held in metadata and it is important for drawing external resources. The process of managing and mining complex procedures is tough for sourcing data. ETL processes help in setting up data for use and in the relationship they make.
Hence, using ETL tools above benefits are achieved for the business. The current ETL tools that businesses are using are MarkLogic, Oracle, Amazon RedShift with a host of new age solutions that offer competitive price packages and services.
Take Away
The ETL process is an important data analytics stack for the extraction, transformation, and loading of data. From multiple sources to the data warehouse. The extraction phase ensures data is extracted from diverse sources at the staging area. While the transformation phase ensured data that is extracted is cleansed and transformed. And finally Loading phase moves the data to the target data warehouse.
While ETL process is an advantage at all steps, as a business you should be careful that: When cleansing data, it is a planned event and there is control on the costs of cleansing and the time it takes to complete the process. Secondly, have a cost strategy wherein you can bring down the storage costs by moving summarized data to disk tapes. Thirdly, ensure volume of data stored and its use has to be leveled-off at data granularity level to lower storage costs.